Table of Contents
Highlights
- On-device AI allows smartphones to process tasks locally, reducing latency, enabling offline functionality, and safeguarding user privacy by minimising cloud transmission.
- From computational photography and health tracking to voice assistants and accessibility tools, on-device AI powers a wide range of intelligent features.
- With continued advancements in both hardware and software, on-device AI is set to drive the next generation of personal and privacy-conscious smart devices.
The transformation of smartphones over the past two decades has redefined modern communication, productivity, and entertainment. At the core of this evolution is Artificial Intelligence, which has transitioned from being a cloud-based novelty to a powerful, localised force within personal devices.
In recent years, the integration of AI directly into personalised devices, known as on-device AI, has emerged as a pivotal development. Unlike traditional cloud-based AI systems that rely on external servers for data processing, on-device AI performs computations locally on the device itself. It delivers significant improvements in speed, security, personalisation, and reliability, transforming the user experience in profound ways.
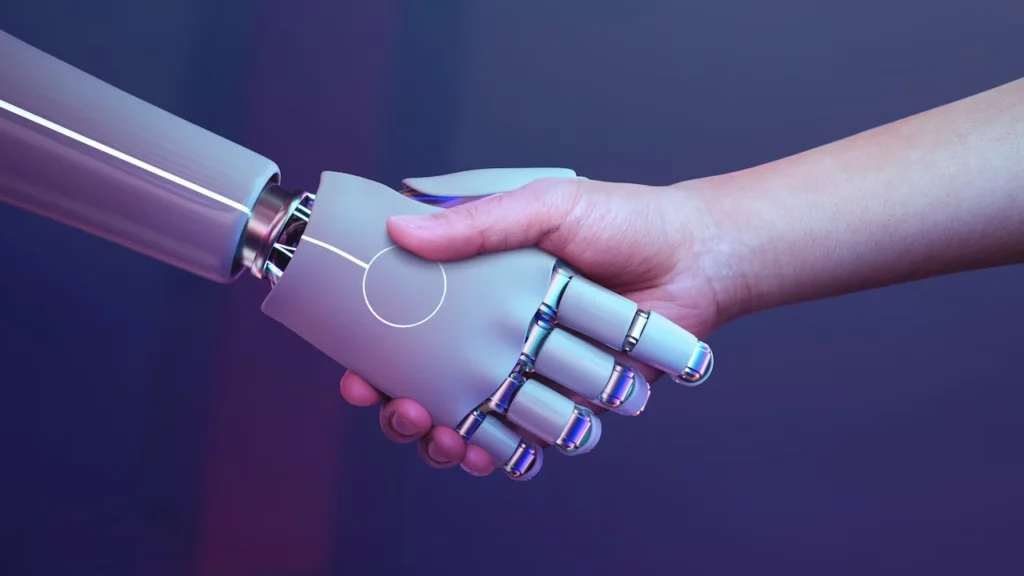
The Shift from Cloud-Based to On-Device AI
Initially, smartphone AI capabilities relied heavily on cloud computing. Voice assistants like Apple’s Siri, Google Assistant, and Amazon’s Alexa processed user queries by transmitting them to remote servers, where the data was analysed and a response was sent back to the user who generated the query. While effective, this method had several limitations, including latency, the requirement of stable internet connectivity, and potential privacy concerns due to the transmission of personal data.
On-device AI addresses these concerns by allowing data to be processed directly on the smartphone. This efficiently minimises latency, enhances data privacy, and enables devices to function effectively even without an internet connection, which is an essential feature in areas with unreliable or limited connectivity. Real-time responsiveness is particularly important for applications such as voice assistants, camera processing, and augmented reality, where delays can negatively impact the user experience.
The shift to on-device AI has been made possible by a combination of software innovation and hardware breakthroughs. Modern mobile processors are now equipped with dedicated components such as Neural Processing Units (NPUs) and AI-optimised GPUs, which are capable of handling complex machine learning tasks efficiently. These accelerators support instantaneous inference of tasks, like facial recognition, natural language processing, and image segmentation, all while maintaining energy efficiency for mobile environments.
The Foundations of On-Device AI
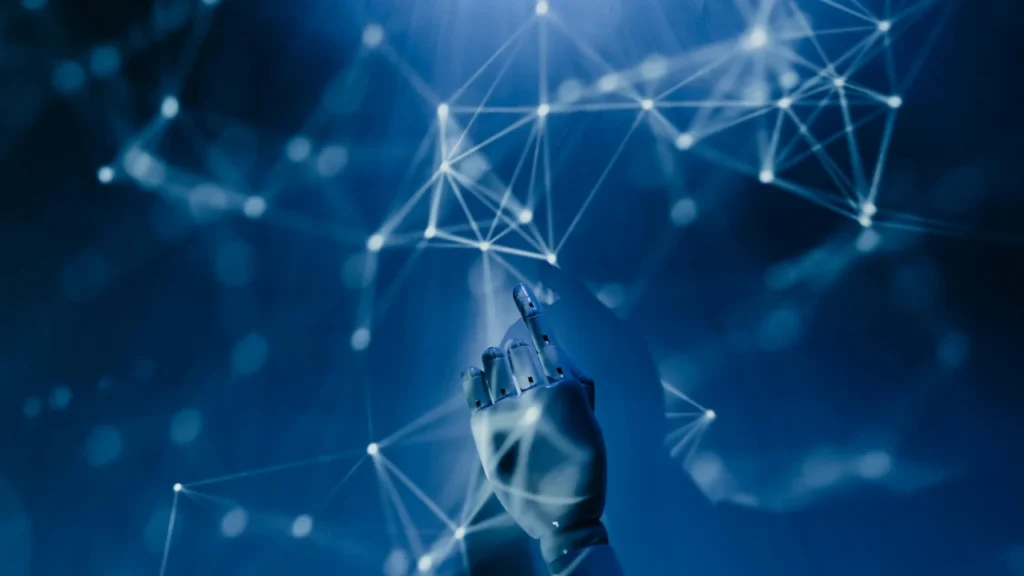
At the heart of on-device AI lies dedicated hardware such as Neural Processing Units (NPUs), Digital Signal Processors (DSPs), and AI-optimised CPUs and GPUs. These components are specifically designed to accelerate AI and machine learning workloads while minimising power consumption. NPUs, in particular, are specially tailored to perform matrix and tensor operations critical to deep learning, enabling real-time execution of tasks such as image classification, object detection, and language processing.
DSPs are optimised for efficient parallel processing and are often used in audio, video, and sensor data analysis. By offloading AI computations to these specialised units, smartphones achieve a balance between high performance and prolonged battery life, which is essential for delivering a smooth and sustainable user experience.
AI-Centric Chipsets Powering Modern Smartphones
The processing power required to run advanced AI models locally has led to a new wave of innovation in mobile chipsets. Companies are now designing System-on-Chips (SoCs) specifically optimised for AI workloads, embedding specialised components that accelerate machine learning tasks and allow smartphones to perform complex functions independent from the cloud.
Qualcomm’s Snapdragon 8 Gen 3 platform, for example, integrates AI directly into the image and video processing pipeline, enabling real-time segmentation, object tracking, and enhanced photography. It also makes use of its Hexagon NPU to facilitate on-device natural language processing and camera-based scene recognition, improving both performance and battery efficiency.
On the other hand, MediaTek’s Dimensity 9400 features the Agentic AI Engine, which supports on-device training and self-evolving AI agents capable of understanding user routines and adapting over time to provide long-term assistance. The chipset also enables language translation and context-aware interaction without relying on a cloud connection.
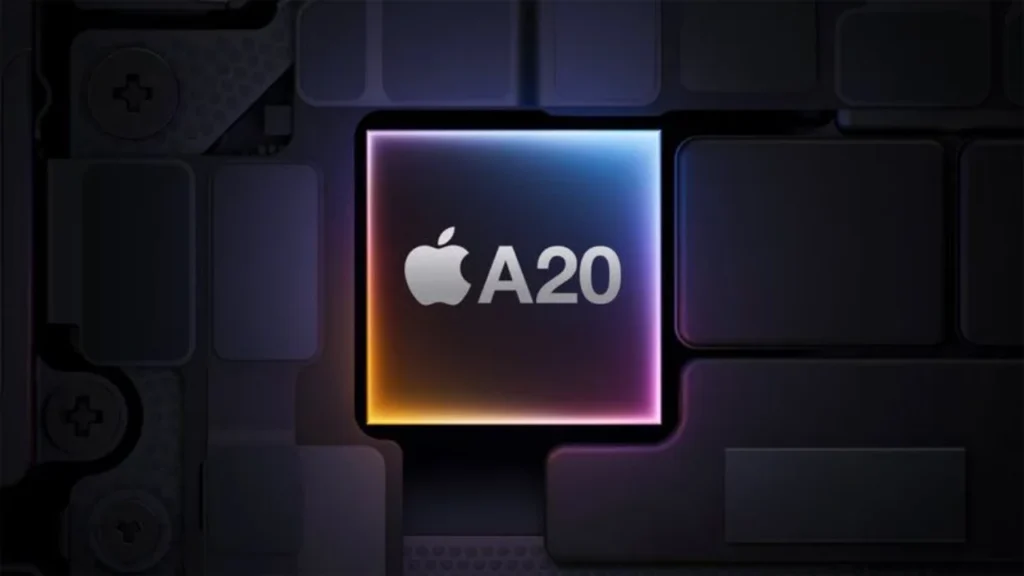
Apple’s A-series chips, especially with the introduction of the Neural Engine starting from the A11 Bionic, have continued to evolve with each iteration, significantly boosting the performance of the available AI-driven features. These include Face ID, Animoji, and the on-device version of Siri, all of which function extremely efficiently without needing to send data to the cloud.
The A17 Pro chip in particular includes an enhanced 16-core Neural Engine capable of performing over 35 trillion operations per second, giving way for even more advanced features like on-the-spot language transcriptions, improved visual analysis, and a personalised user experience that remains private and secure.
Additional Enhancements
Memory and storage are also optimised to support this form of AI technology. Advancements like LPDDR5X RAM and Universal Flash Storage (UFS) 4.0 storage amplify data throughput and reduce latency, which are crucial for AI applications that need to function in real-time. These innovations ensure faster read/write speeds, enabling access to large AI models and datasets stored on the device in a much more efficient manner.
LPDDR5X RAM, for example, delivers significantly higher bandwidth than its predecessors, allowing simultaneous execution of multiple AI tasks without bottlenecks. Meanwhile, UFS 4.0 storage supports rapid app loading, smoother multitasking, and accelerated AI data caching, improving the overall responsiveness of AI features.
Software frameworks such as Apple’s Core ML, Google’s TensorFlow Lite, and Qualcomm’s AI Engine SDK provide developers with the tools to integrate on-device AI functionalities into Apps and services. These frameworks offer model conversion tools, performance optimisation libraries, and support for hardware acceleration, making it easier to deploy machine learning models across a variety of mobile hardware configurations. TensorFlow Lite, for instance, includes delegates for NPUs and GPUs to maximise performance, while Core ML supports seamless integration with iOS features like Vision and Natural Language frameworks. These tools play an important role in bringing cutting-edge AI experiences to users in a more accessible manner.
Real World Applications of On-Device AI
As on-device AI capabilities continue to mature, their practical applications are becoming increasingly diverse and impactful. These AI-driven features are transforming the everyday smartphone experience, whether it is enhancing multimedia capabilities or improving health monitoring, personalisation, or accessibility.
Photography and Videography
On-device AI has dramatically changed modern smartphone photography. Computational photography now makes use of AI to improve image quality right as the image is being taken. Smartphones can now recognise scenes, adjust lighting, apply HDR, and enable features like portrait mode and night sight. All this can be achieved without the need for cloud processing. Google Pixel’s Tensor chip uses on-device AI to process photos, ensuring high-quality results even in poor lighting. Apple’s Photonic Engine and Smart HDR system, on the other hand, uses local machine learning to enhance image details and colour accuracy.
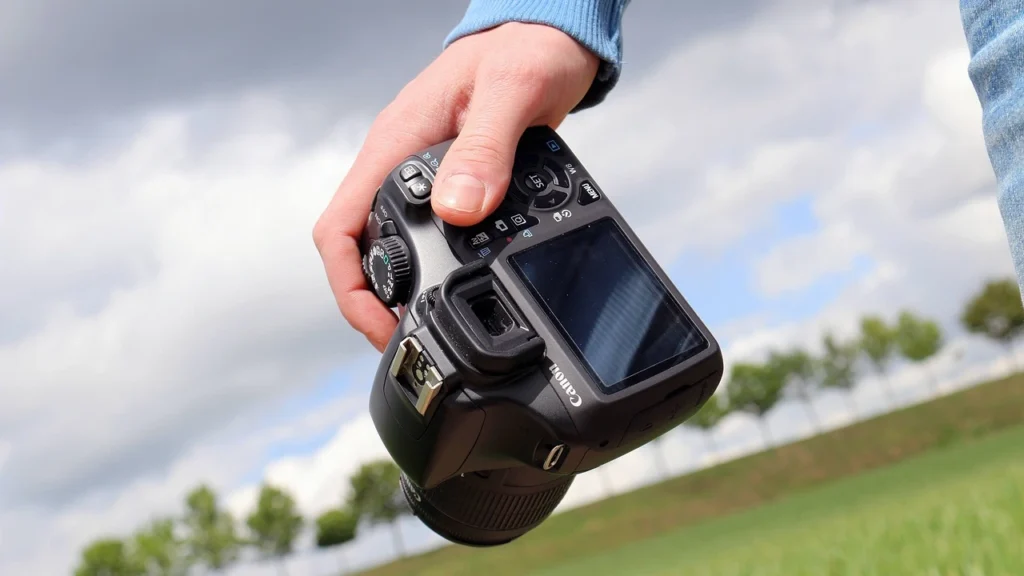
Moreover, these AI technologies go beyond just static image processing. During real-time video recording, benefits such as live AI adjustments for stabilisation, noise reduction, and dynamic exposure controls can be taken advantage of. Some smartphones also make use of AI systems to generate depth maps for improved bokeh effects and to create ultra-detailed panoramas. This level of processing not only saves time by eliminating post-processing needs but also heightens user creativity by offering professional-grade features in a much more accessible interface.
Voice Assistants and Personalisation
Modern voice assistants have become more responsive, thanks to the implementation of on-device AI. Apple’s Siri, for example, now processes many commands directly on the iPhone, reducing response times and consequently increasing reliability. Google Assistant also makes use of AI-driven speech recognition to perform tasks like setting reminders or sending messages, all of which can work without the internet.
Additionally, these systems allow for more nuanced and context-aware interactions. Assistants can now better understand natural language, disambiguate user intent, and remember context from recent queries, all while operating within the confines of the device.
AI also enables smartphones to learn from user behaviour and preferences. AI systems can tailor app recommendations, manage battery usage integrity, prioritise notifications, and adapt settings like screen brightness and volume, all based on user habits. Adaptive battery and brightness on Android devices are prime examples of such features that rely on onboard machine learning to optimise power consumption and usability.
Health and Fitness
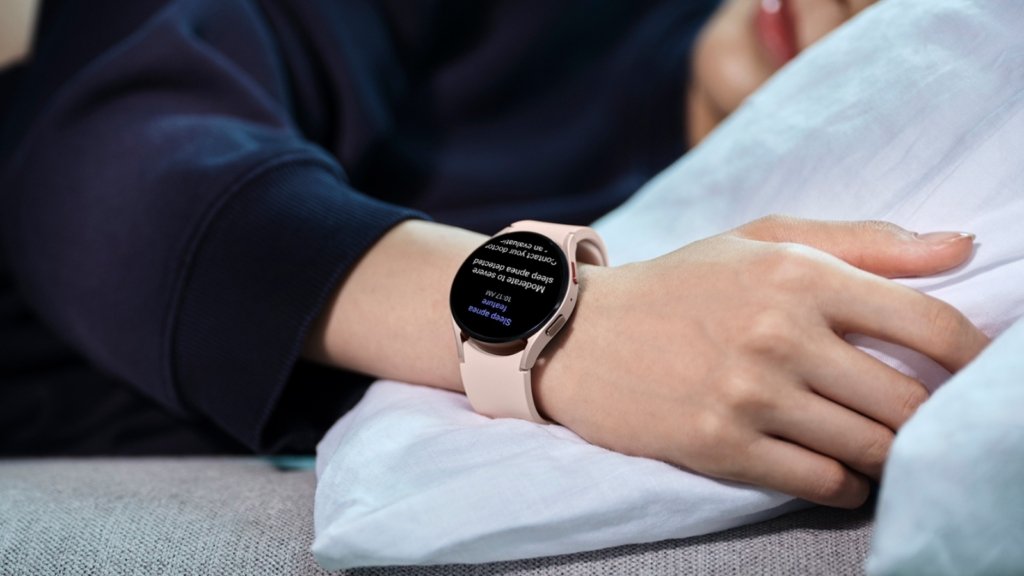
Devices that are better equipped with in-device AI can analyse data from sensors to provide instantaneous insights into physical activities, sleep patterns, and overall health. Apple’s Health app and Google Git use AI to track and interpret health data, powering other systems such as fall detection, heart rate monitoring, and ECG analysis in devices like the Apple Watch and Samsung Galaxy Watch.
In addition to monitoring basic metrics, AI technologies enable advanced predictive analysis for preventive healthcare. For instance, AI can detect irregular heart rhythms or breathing patterns and provide the user with a timely alert before any of the symptoms become severe. This predictive functionality can also be used to monitor trends over time and suggest various lifestyle changes based on long-term health data.
Security and Accessibility
On-device AI plays a pivotal role in enhancing smartphone security. Biometric authentication systems like Face ID and fingerprint scanners use AI to adapt over time, improving accuracy and resilience against spoofing. These systems analyse subtle changes in user behaviour and appearance, such as minor facial feature variations or skin texture differences, to continually refine their matching algorithms.
AI also powers features such as spam call detection, phishing prevention, and anomaly detection in login patterns. For example, machine learning models running locally can identify suspicious patterns in call metadata or email behaviour, flagging them as potentially harmful.
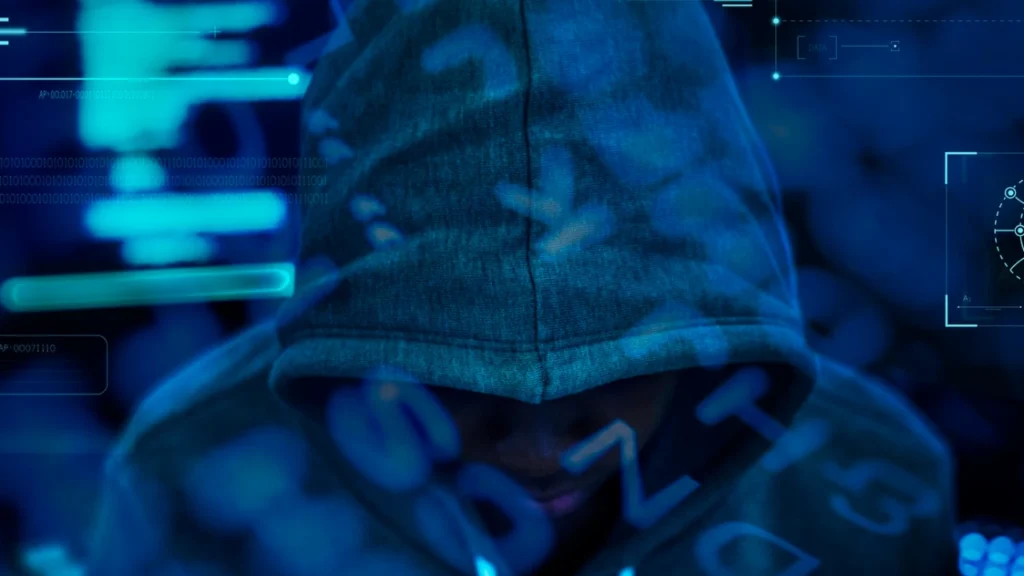
AI-driven features have also greatly improved smartphones when it comes to accessibility. On-device capabilities such as real-time transcription, screen reading, voice control, and image description help users with visual, auditory, or motor impairments. Google’s Lookout and Apple’s VoiceOver use these improvements to identify objects, read text out loud, and navigate interfaces.
Recent innovations also include features like gesture-based control systems and real-time sign language recognition that cater to a wider range of accessibility needs. Additionally, AI-enhanced voice synthesis allows for more natural and customizable speech output for users who rely on text-to-speech, providing a richer and more inclusive interaction with digital devices.
Challenges and Limitations
Despite its many benefits, on-board AI faces potential challenges. Power consumption remains a prime concern, as AI tasks can be demanding. Efficient thermal management and energy optimisation are essential to prevent overheating and battery drain. Model size and storage constraints also limit the complexity of AI that can be run on mobile hardware. Furthermore, fragmentation in hardware capabilities across devices poses difficulties for developers aiming to deliver consistent AI experiences.
Security is another challenge. While on-device processing reduces exposure to cloud-based threats, it requires robust firmware and secure hardware to prevent local exploits. Keeping AI models up to date without compromising security or user control is an ongoing area of research and development. Additionally, the integration of third-party apps and services presents another layer of vulnerability, as not all developers adhere to the same secure protocols. Ensuring that these apps can access on-device AI capabilities without risking exposing sensitivity requires strict access protocols, sandboxing techniques and frequent auditing.
The Future of On-Device AI
To explain simply, we are looking at a promising and expansive future when it comes to onboard AI systems. Advancements in chip design, such as Apple’s Neural Engine, Qualcomm’s Hexagon DSPs, and Google’s Edge TPUs, will enable even more complex and energy-efficient AI applications. These advancements will be the factor that paves the way for AI agents that can plan, reason, and autonomously interact with users across a variety of tasks. In the coming days, we are likely to see smart devices that will anticipate user needs, manage schedules, analyse health trends, provide real-time language coaching, and even respond to emotional cues.